Quantum Entanglement Offers Unprecedented Precision for GPS, Imaging and Beyond
University of Arizona researchers are using quantum entanglement to detect radio frequencies with more sensitivity and accuracy than ever.
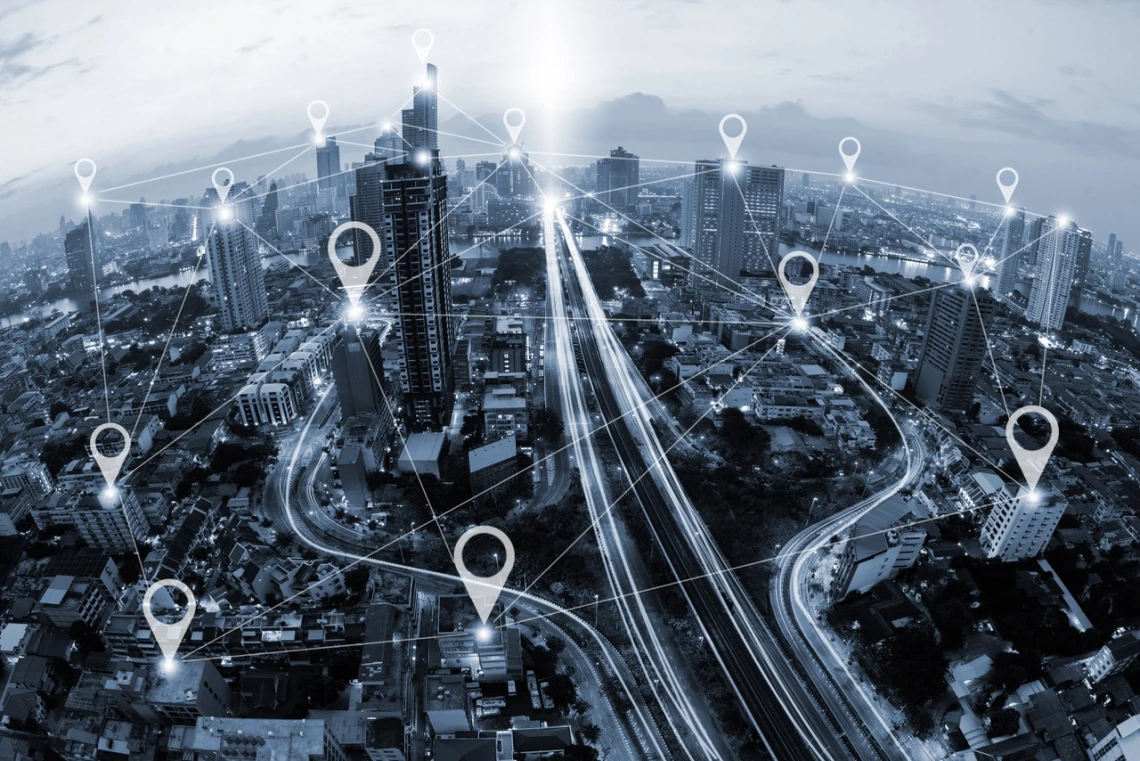
University of Arizona engineers have demonstrated how quantum capabilities could improve functions like GPS, medical imaging, astronomy observation and more.
Your phone’s GPS, the WiFi in your house and communications on aircraft are all powered by radio-frequency waves, or RF waves, which carry information from a transmitter at one point to a sensor at another. The sensors interpret this information in different ways. For example, a GPS sensor determines its location by using the amount of time it takes to receive a signal from a satellite. For applications such as in-door localization and defeating spoofing GPS signals, a wireless sensor measures the angle at which it receives an RF wave. The more precisely the sensor can measure this time delay or angle of arrival, the more it can accurately determine location or enhance security.
In a paper published today in Physical Review Letters, University of Arizona engineering and optical sciences researchers, in collaboration with engineers from General Dynamics Mission Systems, demonstrate how a combination of two techniques -- radio frequency photonics sensing and quantum metrology -- can give sensor networks a previously unheard-of level of precision. The work involves transferring information from electrons to photons, then using quantum entanglement to increase the photons’ sensing capabilities.
“This quantum sensing paradigm could create opportunities to improve GPS systems, astronomy laboratories and biomedical imaging capabilities,” said Zheshen Zhang, assistant professor of materials science and engineering and optical sciences, and principal investigator of the university’s Quantum Information and Materials Group. “It could be used to improve the performance of any application that requires a network of sensors.”
From Electrons to Photons
Traditional antenna sensors transform information from RF signals to an electrical current made up of moving electrons. However, optical sensing, which uses photons, or units of light, to carry information, is much more efficient. Not only can photons hold more data than electrons, giving the signal larger bandwidth, but photonics-based sensing can transmit that signal much farther than electronics-based sensing, and with less interference.
Because optical signals offer so many advantages, the researchers used an electro-optical transducer to convert RF waves into the optical domain in a method called RF-photonics sensing.
“We designed a bridge between an optical system and a physical quantity in a completely different domain,” Zhang explained. “We demonstrated that with an RF domain in this experiment, but the idea could also be applied to other scenarios. For example, if you want to measure temperature using photons, you could use a thermo-optical transducer to convert the temperature into an optical property.”
Entangled Sensors
After converting information to the optical domain, the researchers applied a technique called quantum metrology. Usually, a sensor’s precision is limited by something called the standard quantum limit. For example, smartphone GPS systems are usually accurate within a 16-foot radius. Quantum metrology uses entangled particles to break past the standard quantum limit and take ultrasensitive measurements.
How does it work? Entangled particles are tied together so anything that happens to one particle affects the particles it’s entangled with as well, as long as appropriate measurements are taken.
Picture a supervisor and an employee working together on a project. Because it takes time for the employee to share information with his supervisor through methods like emails and meetings, the efficiency of their partnership is limited. But if the two could entangle their brains together, the employee and the supervisor would automatically have the same information -- saving time and allowing them to jointly tackle a common problem more efficiently.
Quantum metrology has been used to improve sensor precision in places like the Laser Interferometer Gravitational-Wave Observatory, or LIGO, which has opened up a new window for astronomers. However, almost all prior quantum metrology demonstrations, including LIGO, only involve a single sensor.
Connecting Sensor Networks
However, RF waves are usually received by a network of sensors, each of which processes information individually -- more like a group of independent employees working with their supervisors. Quntao Zhuang, UA assistant professor of electrical and computer engineering, previously demonstrated a theoretical framework to boost performance by teaming up entangled sensors.
This new experiment demonstrated for the first time that a network of three sensors can be entangled with one another, meaning they all receive the information from probes and correlate it with one another simultaneously. It’s more like if a group of employees could share information instantly with their bosses, and the bosses could instantly share that information with each other, making their workflow ultra-efficient.
“Typically, in a complex system -- for example, a wireless communications network or even our cellphones -- there’s not just a single sensor, but a set of sensors that work together to undertake a task,” Zhang said. “We’ve developed a technology to entangle these sensors, rather than having them operate individually. They can use their entanglement to ‘talk’ to each other during the sensing period, which can significantly improve sensing performance.”
While the experiment only used three sensors, it opens the door to the possibility of applying the technique to networks of hundreds of sensors
“Imagine, for example, a network for biological sensing: You can entangle these biosensors so that they work together to identify the species of a biological molecule, or to detect neural activities more precisely than a classical sensor array,” Zhang said. “Really, this technique could be applied to any application that requires an array or network of sensors.”
One potential application is in the entangled photon network being built on the University of Arizona campus. In theory work published in Physical Review X in 2019, Zhuang presented how machine learning techniques can train sensors in a large-scale entangled sensor network like this one to take ultra-precise measurements.
“Entanglement allows sensors to more precisely extract features from the parameters being sensed, allowing for better performance in machine learning tasks such as sensor data classification and principal component analysis,” Zhuang said. “Our previous work provides a theoretical design of an entanglement-enhanced machine learning system that outperforms classical systems.”
In addition to Zhang and Zhuang, the paper’s authors were Yi Xia, PhD student in optical sciences; Wei Li, a postdoctoral researcher in the Department of Materials Science and Engineering; and William Clark and Darlene Hart of General Dynamics Mission Systems.