Abhijit Mahalanobis joins highest ranks of inventors as a 2024 NAI fellow
The electrical and computer engineering associate professor is rapidly increasing computing capacities to essentially give eyes to computers.
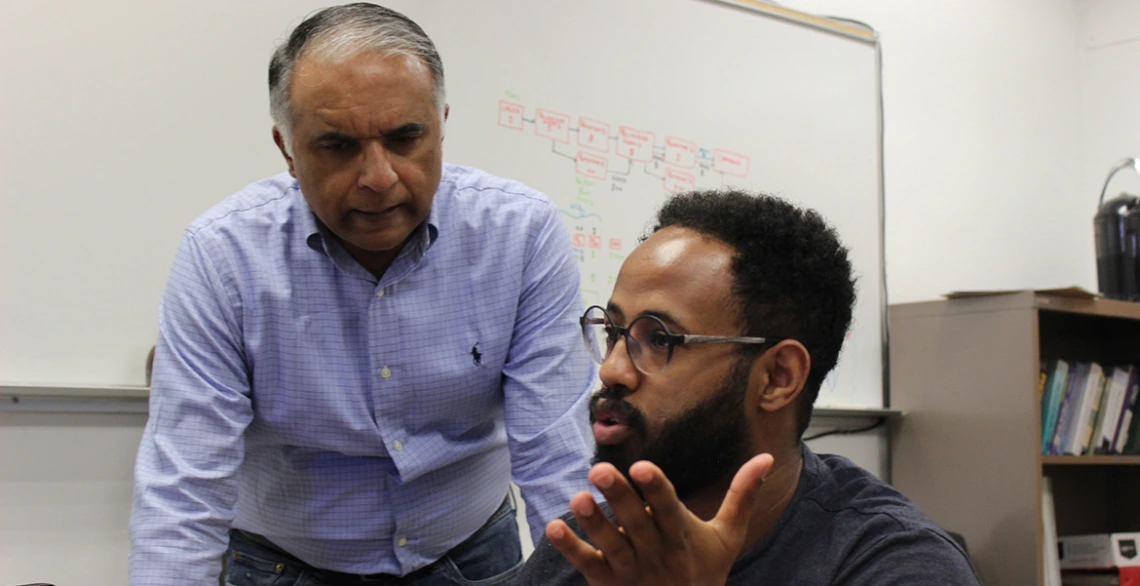
Abhijit Mahalanobis (left) advises doctoral student Natnael Daba. Working with students is rewarding for Mahalanobis, who spent much of his career in industry.
The National Academy of Inventors elected Abhijit Mahalanobis, associate professor of electrical and computer engineering, to its 2024 Class of Fellows, citing his spirit of innovation and dedication to advancing technology.
“I’m very honored, and astonished, to be recognized, given the number of people working in this field and their contributions,” he said.
In his four-decade career, Mahalanobis has made extensive contributions to an area of computer vision called correlation pattern recognition. This sophisticated type of artificial intelligence allows computers to analyze vast amounts of visual data and is applied to diverse fields, from medical diagnosis to defense.
“It’s truly inspiring to see Abhijit’s work receive this level of acknowledgment,” said Michael Wu, ECE department head and Kenneth Von Behren Endowed Professor. “This prestigious honor is a testament to his remarkable achievements.”
NAI fellowship is the highest professional distinction awarded solely to inventors, according to the academy. Mahalanobis has joined a group whose careers are celebrated with honors and distinctions such as the Nobel Prize, U.S. National Medal of Technology and Innovation and National Medal of Science.
At the forefront of recognition methods
Kumar Bhagavatula is a professor of electrical and computer engineering at Carnegie Mellon University who endorsed Mahalanobis’ selection.
“Abhijit has been at the forefront of developing and deploying pattern recognition – now more popularly known as machine learning – algorithmic inventions for challenging applications such as automatic target recognition, object detection and tracking,” said Bhagavatula.
Computers use target recognition to interpret scenes or distinguish different objects from an image. Correlation pattern recognition examines changes in lightness or darkness across the object’s surface, called spatial frequencies, from light waves reflected off a target object.
“Think about the shape of an apple being made up of these undulating black-and-white patterns that mix. And when they add up together, they re-construct the shape of an apple,” Mahalanobis said.
Interference patterns play an important role in correlation pattern recognition as well.
“If you drop pebbles into water, the resulting waves have amplitudes that are adding or subtracting. Spatial frequencies are much the same, but in terms of bright and dark intensities. The way they come together can create the shapes we see in the images.”
Correlation pattern recognition and the spatial frequency techniques that Mahalanobis has developed have been critical for defense applications, for instance in making target recognition and tracking of distant objects more accurate. Mahalanobis' spatial frequency innovations could be used in future computer vision applications, which are increasingly based on deep learning, or neural networks.
"I believe frequency domain techniques have unexplored potential to play a role in making today's advanced methods more computationally efficient, and able to learn with less data," said Mahalanobis. "This is important for fields where there are computing constraints, such as in medical applications of machine vision, self-driving capabilities in vehicles, or in small portable devices.”
Career accomplishments abound
After earning his bachelor’s degree at the University of California, Santa Barbara, Mahalanobis completed master’s and doctoral degrees at Carnegie Mellon University. While there, he invented the minimum average correlation energy (MACE) filter, the first correlation filter for the frequency domain, and led the way in finding new methods to improve target recognition systems.
Mahalonobis’ trailblazing spirit led to his election as a fellow of the Institute of Electrical and Electronics Engineers, the Optical Society of America (now known as Optica), and the Society of Photographic Instrumentation Engineers. The State of Arizona named him Innovator of the Year as part of the Governor’s Awards in 2000, and Science Spectrum selected him as Scientist of the Year in 2006.
Mahalanobis holds six patents for his technological image recognition and processing designs, has authored more than 190 articles and five book chapters, and co-authored the definitive book on correlation pattern recognition. In addition to his service in academia, Mahalanobis worked in industry, for Lockheed Martin and Raytheon, an RTX Business, for more than 25 years. He joined the College of Engineering in 2022.
“I feel like I stand on the shoulders of giants, and I’m grateful if my inventions have made a difference,” said Mahalanobis. “Here at the university, my goal is to take whatever I’ve learned out there in the real world, pass that on, and encourage creativity in our students.”
The NAI will formally induct Mahalanobis at a ceremony in Atlanta in June 2025, together with fellow U of A professor Shibin Jiang.